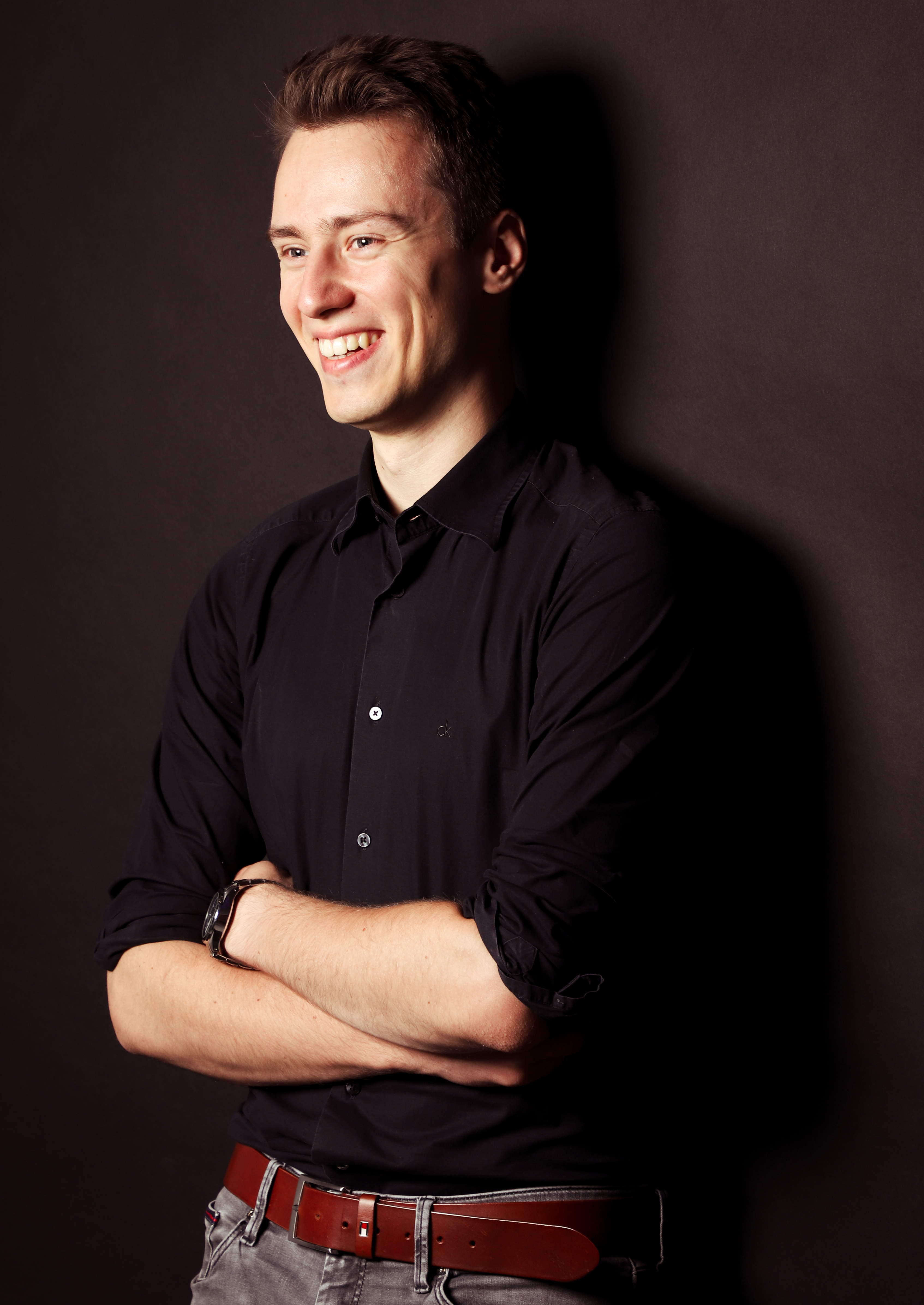
Office 20-30/A21
Maria-von-Linden-Str. 6
D-72076 Tübingen
Marvin Pförtner
I am a PhD student in Philipp Hennig’s group at the University of Tübingen and the International Max Planck Research School for Intelligent Systems (IMPRS-IS). My research interests lie at the intersection of Bayesian machine learning and numerical analysis. More specifically, my work revolves around
- algorithms for scalable (approximate) Gaussian process inference,
- Gaussian process theory (sample path properties, Gaussian measure theory),
- probabilistic numerical methods for partial differential equations, and
- Bayesian deep learning with Laplace approximations.
I’m also interested in applications of all the above to scientific inference tasks.
I like to tackle problems using the framework of matrix-free (probabilistic) numerical linear algebra, which often leads to elegant and efficient algorithms.
news
Mar 12, 2025 | I will present our work on Computation-Aware Kalman Filtering and Smoothing at AISTATS 2025 in Mai Khao, Thailand. |
---|---|
Jan 2, 2025 | I will give a talk on “Probabilistic Functional Programming” at the MFO Workshop 2505 on “Overparametrization, Regularization, Identifiability and Uncertainty in Machine Learning” in Oberwolfach, Germany. |
Dec 1, 2024 | I will be attending NeurIPS 2024 in Vancouver. |
selected publications
- In Advances in Neural Information Processing Systems, 2024